The Learning System Model in Machine Learning: A Comprehensive Guide
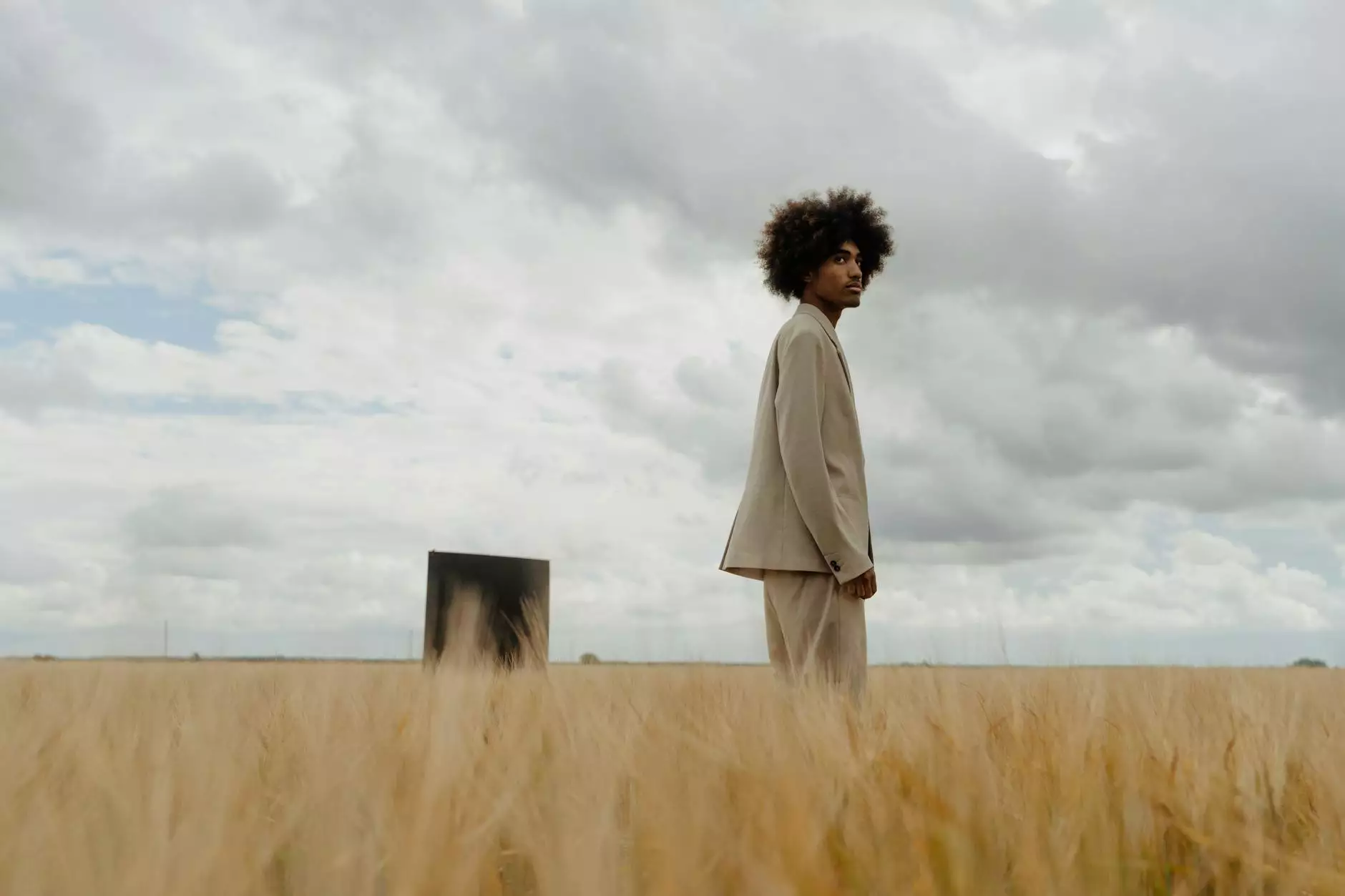
In the rapidly evolving world of technology, the learning system model in machine learning serves as a cornerstone for organizations seeking to leverage data-driven strategies to enhance operational efficiency and gain a competitive edge. Understanding this model is crucial for businesses aiming to implement effective content management services, streamline business process automation services, and establish robust data governance systems.
Understanding Machine Learning
Machine learning (ML) is a subset of artificial intelligence (AI) that enables systems to learn from data and improve their performance over time without being explicitly programmed. This transformative technology has broad applications across various sectors, including finance, healthcare, marketing, and more. The principles of machine learning are grounded in algorithms and statistical models that identify patterns in large datasets.
The Importance of Learning System Models
The learning system model in machine learning serves as a framework for understanding how machines acquire knowledge from data. This model can dramatically enhance business capabilities by:
- Improving Decision-Making: Algorithms analyze historical data to provide insights that drive informed decisions.
- Enhancing Customer Experience: Predictive analytics enables personalized offerings, fostering customer loyalty.
- Automating Processes: Streamlined workflows reduce human error and free up resources for strategic tasks.
Components of a Learning System Model
The learning system model comprises several key components that work collaboratively to ensure effective learning:
1. Data Input
The foundation of any learning system is the data it processes. Data can come from various sources, including:
- Transactional data
- User behavior data
- Sensor data from IoT devices
Collecting high-quality, relevant data is essential for training machine learning models and obtaining valuable outputs.
2. Learning Algorithms
Learning algorithms are at the heart of the learning system model in machine learning. Common types of algorithms include:
- Supervised Learning: Models learn from labeled data to predict outcomes.
- Unsupervised Learning: Algorithms identify patterns in unlabeled data, often used for clustering and association.
- Reinforcement Learning: Systems learn optimal actions through trial and error to maximize cumulative rewards.
3. Model Structure
Determining the architecture of a model—whether it is linear, tree-based, or neural networks—is critical for handling specific tasks. The structure influences the model's capacity to generalize from training data to make accurate predictions on new data.
4. Output Generation
The generated output from the model serves as actionable insights or predictions that can guide business strategies. Outputs can range from simple categorizations to complex recommendations based on predictive analysis.
5. Feedback Loop
A vital element of the learning system model is the feedback loop, which allows for continuous improvement. By integrating new data, the model refines its predictions over time, adapting to changes in business environments and consumer behavior.
Applications of Learning System Models in Business
The applicability of the learning system model in machine learning in business is extensive. Here are some prime examples:
Content Management Services
In the realm of content management, machine learning can significantly enhance document classification and content discovery through natural language processing (NLP) algorithms. This leads to:
- Automated Tagging and Categorization: Content can be automatically tagged based on its relevance, making it easier for users to find information.
- Content Optimization: Analyzing user engagement data allows businesses to optimize content for improved user experiences.
Business Process Automation Services
Machine learning algorithms can streamline business processes by automating repetitive tasks and optimizing workflows. Benefits include:
- Increased Efficiency: Automating mundane tasks allows employees to focus on higher-value activities.
- Predictive Maintenance: ML models can predict equipment failures, reducing downtime and operational costs.
Data Governance Systems
Implementing machine learning in data governance helps ensure that data quality and compliance are met through:
- Anomaly Detection: Algorithms can identify and flag data inconsistencies or errors within large datasets.
- Regulatory Compliance: Machine learning can automate reporting processes, ensuring businesses adhere to required regulations.
Challenges and Considerations
While the learning system model in machine learning provides numerous advantages, it also presents challenges that businesses must navigate:
Data Privacy and Security
With stringent regulations surrounding data privacy (e.g., GDPR), organizations must ensure that their data management practices comply with legal standards while leveraging machine learning technologies.
Model Interpretability
As machine learning models become increasingly complex, understanding how they make decisions can become challenging. Ensuring model explainability is crucial for building trust among stakeholders.
Quality of Data
The effectiveness of a learning system model largely depends on the quality of the data used. Organizations must prioritize data collection and governance practices to mitigate biases and inaccuracies.
Future Trends in Learning System Models
The future of the learning system model in machine learning is poised for exciting advancements:
Federated Learning
Federated learning allows for decentralized model training across multiple devices without sharing sensitive data, which enhances privacy while still benefiting from collective insights.
Transfer Learning
This approach enables models to apply knowledge gained in one domain to another, accelerating the training process and improving performance even with limited data.
Explainable AI (XAI)
As businesses strive for transparency, XAI focuses on making machine learning models more interpretable, helping end-users understand the reasoning behind model predictions.
Conclusion
In summary, the learning system model in machine learning is not just a technological gimmick but a transformative approach that can redefine how businesses operate. By leveraging machine learning, companies can enhance their content management services, optimize business process automation services, and establish comprehensive data governance systems. Though challenges exist, the benefits greatly outweigh them when businesses implement these systems thoughtfully and strategically. The future of machine learning in business promises continued innovations, making it an exciting time to invest in this technology.
To take full advantage of these advancements, businesses must embrace the learning system model in machine learning as a critical component of their strategic roadmap. As a partner in this journey, Intalio offers unrivaled expertise in machine learning applications tailored for your organization's needs.